Note
Click here to download the full example code
Random Search vs. Bayesian Optimization¶
In this section, we demonstrate the behaviors of random search and Bayesian optimization in a simple simulation environment.
Create a Reward Function for Toy Experiments¶
Import the packages:
import numpy as np
import matplotlib.pyplot as plt
from mpl_toolkits.mplot3d import Axes3D
Generate the simulated reward as a mixture of 2 gaussians:
Input Space x = [0: 99], y = [0: 99]. The rewards is a combination of 2 gaussians as shown in the following figure:
def gaussian2d(x, y, x0, y0, xalpha, yalpha, A):
return A * np.exp( -((x-x0)/xalpha)**2 -((y-y0)/yalpha)**2)
x, y = np.linspace(0, 99, 100), np.linspace(0, 99, 100)
X, Y = np.meshgrid(x, y)
Z = np.zeros(X.shape)
ps = [(20, 70, 35, 40, 1),
(80, 40, 20, 20, 0.7)]
for p in ps:
Z += gaussian2d(X, Y, *p)
Visualize the reward space:
fig = plt.figure()
ax = fig.gca(projection='3d')
ax.plot_surface(X, Y, Z, cmap='plasma')
ax.set_zlim(0,np.max(Z)+2)
plt.show()
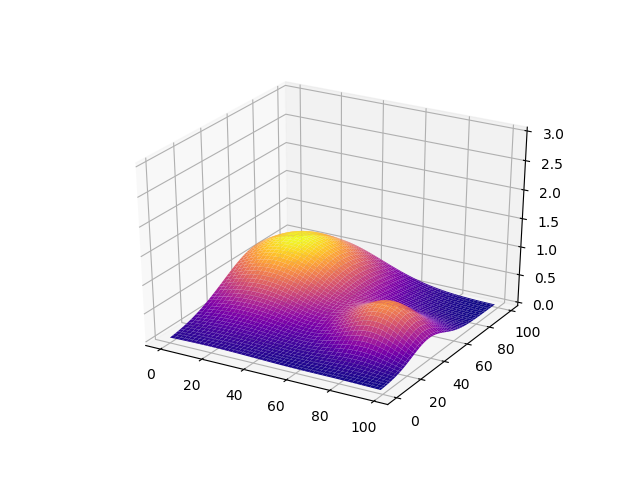
Create Training Function¶
We can simply define an AutoTorch searchable function with a decorator at.gargs. The reporter is used to communicate with AutoTorch search and scheduling algorithms.
import autotorch as at
@at.args(
x=at.Int(0, 99),
y=at.Int(0, 99),
)
def toy_simulation(args, reporter):
x, y = args.x, args.y
reporter(accuracy=Z[y][x])
Random Search¶
random_scheduler = at.scheduler.FIFOScheduler(toy_simulation,
resource={'num_cpus': 1, 'num_gpus': 0},
num_trials=30,
reward_attr="accuracy",
resume=False)
random_scheduler.run()
random_scheduler.join_jobs()
print('Best config: {}, best reward: {}'.format(random_scheduler.get_best_config(), random_scheduler.get_best_reward()))
Out:
Best config: {'x': 22, 'y': 72}, best reward: 0.9942633286025881
Bayesian Optimization¶
bayesopt_scheduler = at.scheduler.FIFOScheduler(toy_simulation,
searcher='bayesopt',
resource={'num_cpus': 1, 'num_gpus': 0},
num_trials=30,
reward_attr="accuracy",
resume=False)
bayesopt_scheduler.run()
bayesopt_scheduler.join_jobs()
print('Best config: {}, best reward: {}'.format(bayesopt_scheduler.get_best_config(), bayesopt_scheduler.get_best_reward()))
Out:
Best config: {'x': 20, 'y': 70}, best reward: 1.000009105108358
Compare the performance¶
Get the result history:
results_bayes = [v[0]['accuracy'] for v in bayesopt_scheduler.training_history.values()]
results_random = [v[0]['accuracy'] for v in random_scheduler.training_history.values()]
fig = plt.figure()
plt.plot(range(len(results_random)), results_random, range(len(results_bayes)), results_bayes)
plt.show()
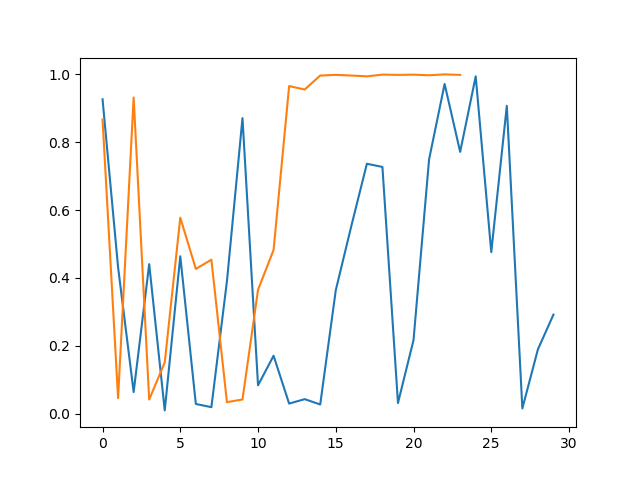
Advance Usage for Bayesian Optimization¶
For some special cases, not all configurations are valid for the requirement. Instead of falling back to random search, we can pre-generate a set of valid configurations using random search, and accelerate the HPO using Bayesian Optimization. The key idea is fitting GP model using observed data points, and using acqusition function to re-rank the pending configurations.
Define valid condiction
We require x or y to be an even number
def is_valid_config(config):
return config['x'] % 2 == 0 or config['y'] % 2 == 0
Pre-generate configurations using random searcher
random_searcher = at.searcher.RandomSearcher(toy_simulation.cs)
lazy_configs = []
valid_cnt = 0
while valid_cnt < 500:
config = random_searcher.get_config()
if is_valid_config(config):
valid_cnt += 1
lazy_configs.append(config)
Initialize lazy configurations with Bayesian optimization
lazy_bayes = at.searcher.BayesOptSearcher(toy_simulation.cs, lazy_configs=lazy_configs)
lazy_scheduler = at.scheduler.FIFOScheduler(toy_simulation,
searcher=lazy_bayes,
resource={'num_cpus': 1, 'num_gpus': 0},
num_trials=20,
reward_attr="accuracy",
resume=False)
lazy_scheduler.run()
lazy_scheduler.join_jobs()
print('Best config: {}, best reward: {}'.format(lazy_scheduler.get_best_config(), lazy_scheduler.get_best_reward()))
Out:
Best config: {'x': 20, 'y': 73}, best reward: 0.9943964671954428
Plot the training curve
fig = plt.figure()
results_lazy = [v[0]['accuracy'] for v in lazy_scheduler.training_history.values()]
plt.plot(range(len(results_lazy)), results_lazy)
plt.show()
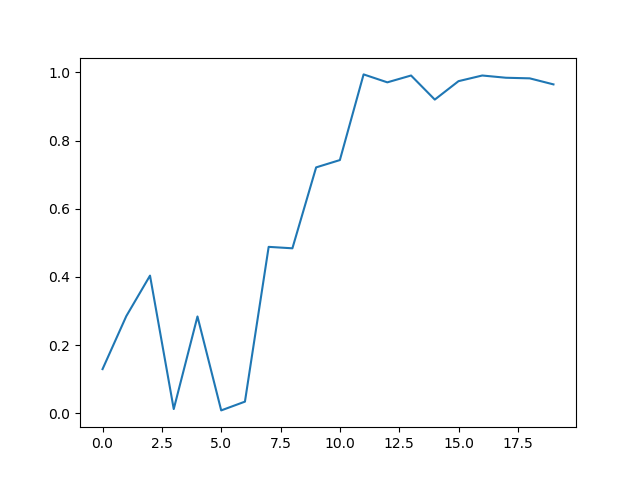
Total running time of the script: ( 0 minutes 17.013 seconds)